Guarda ora Questo tutorial ha un corso video correlato creato dal team di Real Python. Guardalo insieme al tutorial scritto per approfondire la tua comprensione:Lettura e scrittura di file con i panda
Panda è un pacchetto Python potente e flessibile che ti consente di lavorare con dati etichettati e di serie temporali. Fornisce inoltre metodi statistici, abilita la stampa e altro ancora. Una caratteristica cruciale di Pandas è la sua capacità di scrivere e leggere Excel, CSV e molti altri tipi di file. Funziona come i Panda read_csv()
il metodo ti consente di lavorare con i file in modo efficace. Puoi usarli per salvare i dati e le etichette dagli oggetti Pandas in un file e caricarli in seguito come Pandas Series
o DataFrame
istanze.
In questo tutorial imparerai:
- Quali sono gli strumenti Pandas IO L'API è
- Come leggere e scrivere dati da e verso i file
- Come lavorare con vari formati di file
- Come lavorare con i big data efficiente
Iniziamo a leggere e scrivere file!
Bonus gratuito: 5 pensieri su Python Mastery, un corso gratuito per sviluppatori Python che ti mostra la roadmap e la mentalità di cui avrai bisogno per portare le tue abilità in Python al livello successivo.
Installazione di Panda
Il codice in questo tutorial viene eseguito con CPython 3.7.4 e Pandas 0.25.1. Sarebbe utile assicurarsi di avere le ultime versioni di Python e Pandas sulla tua macchina. Potresti voler creare un nuovo ambiente virtuale e installare le dipendenze per questo tutorial.
Innanzitutto, avrai bisogno della libreria Pandas. Potresti averlo già installato. In caso contrario, puoi installarlo con pip:
$ pip install pandas
Una volta completato il processo di installazione, Pandas dovrebbe essere installato e pronto.
Anaconda è un'eccellente distribuzione Python fornita con Python, molti pacchetti utili come Pandas e un gestore di pacchetti e ambienti chiamato Conda. Per ulteriori informazioni su Anaconda, consulta Configurazione di Python per Machine Learning su Windows.
Se non hai Panda nel tuo ambiente virtuale, puoi installarlo con Conda:
$ conda install pandas
Conda è potente in quanto gestisce le dipendenze e le loro versioni. Per saperne di più su come lavorare con Conda, puoi consultare la documentazione ufficiale.
Preparazione dei dati
In questo tutorial utilizzerai i dati relativi a 20 paesi. Ecco una panoramica dei dati e delle fonti con cui lavorerai:
-
Paese è indicato dal nome del paese. Ogni paese è nella lista dei primi 10 per popolazione, area o prodotto interno lordo (PIL). Le etichette di riga per il set di dati sono i codici paese di tre lettere definiti nella ISO 3166-1. L'etichetta della colonna per il set di dati è
COUNTRY
. -
Popolazione è espresso in milioni. I dati provengono da un elenco di paesi e dipendenze per popolazione su Wikipedia. L'etichetta della colonna per il set di dati è
POP
. -
Area è espresso in migliaia di chilometri quadrati. I dati provengono da un elenco di paesi e dipendenze per area su Wikipedia. L'etichetta della colonna per il set di dati è
AREA
. -
Prodotto interno lordo è espresso in milioni di dollari USA, secondo i dati delle Nazioni Unite per il 2017. Puoi trovare questi dati nell'elenco dei paesi per PIL nominale su Wikipedia. L'etichetta della colonna per il set di dati è
GDP
. -
Continente è Africa, Asia, Oceania, Europa, Nord America o Sud America. Puoi trovare queste informazioni anche su Wikipedia. L'etichetta della colonna per il set di dati è
CONT
. -
Giornata dell'indipendenza è una data che commemora l'indipendenza di una nazione. I dati provengono dall'elenco dei giorni dell'indipendenza nazionale su Wikipedia. Le date sono visualizzate nel formato ISO 8601. Le prime quattro cifre rappresentano l'anno, i due numeri successivi il mese e gli ultimi due il giorno del mese. L'etichetta della colonna per il set di dati è
IND_DAY
.
Ecco come appaiono i dati come una tabella:
PAESE | POP | AREA | PIL | CONT | IND_DAY | |
---|---|---|---|---|---|---|
CHN | Cina | 1398.72 | 9596.96 | 12234.78 | Asia | |
IND | India | 1351.16 | 3287.26 | 2575.67 | Asia | 15-08-1947 |
Stati Uniti | Stati Uniti | 329,74 | 9833.52 | 19485.39 | N.America | 04-07-1776 |
IDN | Indonesia | 268.07 | 1910.93 | 1015.54 | Asia | 17-08-1945 |
BRA | Brasile | 210.32 | 8515.77 | 2055.51 | S.America | 07-09-1822 |
PAK | Pakistan | 205,71 | 881.91 | 302.14 | Asia | 14-08-1947 |
NGA | Nigeria | 200,96 | 923,77 | 375,77 | Africa | 01-10-1960 |
BGD | Bangladesh | 167.09 | 147,57 | 245,63 | Asia | 26-03-1971 |
RUS | Russia | 146,79 | 17098.25 | 1530,75 | 12-06-1992 | |
MEX | Messico | 126,58 | 1964.38 | 1158.23 | N.America | 16-09-1810 |
Giappone | Giappone | 126.22 | 377,97 | 4872.42 | Asia | |
DEU | Germania | 83.02 | 357.11 | 3693.20 | Europa | |
FRA | Francia | 67.02 | 640.68 | 2582.49 | Europa | 14-07-1789 |
GBR | Regno Unito | 66.44 | 242,50 | 2631.23 | Europa | |
ITA | Italia | 60.36 | 301.34 | 1943.84 | Europa | |
ARG | Argentina | 44,94 | 2780.40 | 637.49 | S.America | 09-07-1816 |
DZA | Algeria | 43.38 | 2381.74 | 167,56 | Africa | 05-07-1962 |
CAN | Canada | 37.59 | 9984.67 | 1647.12 | N.America | 01-07-1867 |
AUS | Australia | 25.47 | 7692.02 | 1408.68 | Oceania | |
KAZ | Kazakistan | 18.53 | 2724,90 | 159,41 | Asia | 16-12-1991 |
Potresti notare che alcuni dati mancano. Ad esempio, il continente per la Russia non è specificato perché si estende sia in Europa che in Asia. Mancano anche diversi giorni di indipendenza perché l'origine dati li omette.
Puoi organizzare questi dati in Python usando un dizionario nidificato:
data = {
'CHN': {'COUNTRY': 'China', 'POP': 1_398.72, 'AREA': 9_596.96,
'GDP': 12_234.78, 'CONT': 'Asia'},
'IND': {'COUNTRY': 'India', 'POP': 1_351.16, 'AREA': 3_287.26,
'GDP': 2_575.67, 'CONT': 'Asia', 'IND_DAY': '1947-08-15'},
'USA': {'COUNTRY': 'US', 'POP': 329.74, 'AREA': 9_833.52,
'GDP': 19_485.39, 'CONT': 'N.America',
'IND_DAY': '1776-07-04'},
'IDN': {'COUNTRY': 'Indonesia', 'POP': 268.07, 'AREA': 1_910.93,
'GDP': 1_015.54, 'CONT': 'Asia', 'IND_DAY': '1945-08-17'},
'BRA': {'COUNTRY': 'Brazil', 'POP': 210.32, 'AREA': 8_515.77,
'GDP': 2_055.51, 'CONT': 'S.America', 'IND_DAY': '1822-09-07'},
'PAK': {'COUNTRY': 'Pakistan', 'POP': 205.71, 'AREA': 881.91,
'GDP': 302.14, 'CONT': 'Asia', 'IND_DAY': '1947-08-14'},
'NGA': {'COUNTRY': 'Nigeria', 'POP': 200.96, 'AREA': 923.77,
'GDP': 375.77, 'CONT': 'Africa', 'IND_DAY': '1960-10-01'},
'BGD': {'COUNTRY': 'Bangladesh', 'POP': 167.09, 'AREA': 147.57,
'GDP': 245.63, 'CONT': 'Asia', 'IND_DAY': '1971-03-26'},
'RUS': {'COUNTRY': 'Russia', 'POP': 146.79, 'AREA': 17_098.25,
'GDP': 1_530.75, 'IND_DAY': '1992-06-12'},
'MEX': {'COUNTRY': 'Mexico', 'POP': 126.58, 'AREA': 1_964.38,
'GDP': 1_158.23, 'CONT': 'N.America', 'IND_DAY': '1810-09-16'},
'JPN': {'COUNTRY': 'Japan', 'POP': 126.22, 'AREA': 377.97,
'GDP': 4_872.42, 'CONT': 'Asia'},
'DEU': {'COUNTRY': 'Germany', 'POP': 83.02, 'AREA': 357.11,
'GDP': 3_693.20, 'CONT': 'Europe'},
'FRA': {'COUNTRY': 'France', 'POP': 67.02, 'AREA': 640.68,
'GDP': 2_582.49, 'CONT': 'Europe', 'IND_DAY': '1789-07-14'},
'GBR': {'COUNTRY': 'UK', 'POP': 66.44, 'AREA': 242.50,
'GDP': 2_631.23, 'CONT': 'Europe'},
'ITA': {'COUNTRY': 'Italy', 'POP': 60.36, 'AREA': 301.34,
'GDP': 1_943.84, 'CONT': 'Europe'},
'ARG': {'COUNTRY': 'Argentina', 'POP': 44.94, 'AREA': 2_780.40,
'GDP': 637.49, 'CONT': 'S.America', 'IND_DAY': '1816-07-09'},
'DZA': {'COUNTRY': 'Algeria', 'POP': 43.38, 'AREA': 2_381.74,
'GDP': 167.56, 'CONT': 'Africa', 'IND_DAY': '1962-07-05'},
'CAN': {'COUNTRY': 'Canada', 'POP': 37.59, 'AREA': 9_984.67,
'GDP': 1_647.12, 'CONT': 'N.America', 'IND_DAY': '1867-07-01'},
'AUS': {'COUNTRY': 'Australia', 'POP': 25.47, 'AREA': 7_692.02,
'GDP': 1_408.68, 'CONT': 'Oceania'},
'KAZ': {'COUNTRY': 'Kazakhstan', 'POP': 18.53, 'AREA': 2_724.90,
'GDP': 159.41, 'CONT': 'Asia', 'IND_DAY': '1991-12-16'}
}
columns = ('COUNTRY', 'POP', 'AREA', 'GDP', 'CONT', 'IND_DAY')
Ogni riga della tabella è scritta come un dizionario interno le cui chiavi sono i nomi delle colonne ei valori sono i dati corrispondenti. Questi dizionari vengono quindi raccolti come valori nei data
esterni dizionario. Le chiavi corrispondenti per data
sono i codici paese di tre lettere.
Puoi usare questi data
per creare un'istanza di un Pandas DataFrame
. Innanzitutto, devi importare Panda:
>>> import pandas as pd
Ora che hai i Panda importati, puoi utilizzare il DataFrame
costruttore e data
per creare un DataFrame
oggetto.
data
è organizzato in modo tale che i codici paese corrispondano a colonne. Puoi invertire le righe e le colonne di un DataFrame
con la proprietà .T
:
>>> df = pd.DataFrame(data=data).T
>>> df
COUNTRY POP AREA GDP CONT IND_DAY
CHN China 1398.72 9596.96 12234.8 Asia NaN
IND India 1351.16 3287.26 2575.67 Asia 1947-08-15
USA US 329.74 9833.52 19485.4 N.America 1776-07-04
IDN Indonesia 268.07 1910.93 1015.54 Asia 1945-08-17
BRA Brazil 210.32 8515.77 2055.51 S.America 1822-09-07
PAK Pakistan 205.71 881.91 302.14 Asia 1947-08-14
NGA Nigeria 200.96 923.77 375.77 Africa 1960-10-01
BGD Bangladesh 167.09 147.57 245.63 Asia 1971-03-26
RUS Russia 146.79 17098.2 1530.75 NaN 1992-06-12
MEX Mexico 126.58 1964.38 1158.23 N.America 1810-09-16
JPN Japan 126.22 377.97 4872.42 Asia NaN
DEU Germany 83.02 357.11 3693.2 Europe NaN
FRA France 67.02 640.68 2582.49 Europe 1789-07-14
GBR UK 66.44 242.5 2631.23 Europe NaN
ITA Italy 60.36 301.34 1943.84 Europe NaN
ARG Argentina 44.94 2780.4 637.49 S.America 1816-07-09
DZA Algeria 43.38 2381.74 167.56 Africa 1962-07-05
CAN Canada 37.59 9984.67 1647.12 N.America 1867-07-01
AUS Australia 25.47 7692.02 1408.68 Oceania NaN
KAZ Kazakhstan 18.53 2724.9 159.41 Asia 1991-12-16
Ora hai il tuo DataFrame
oggetto popolato con i dati su ciascun paese.
Nota: Puoi usare .transpose()
invece di .T
per invertire le righe e le colonne del set di dati. Se usi .transpose()
, quindi puoi impostare il parametro facoltativo copy
per specificare se si desidera copiare i dati sottostanti. Il comportamento predefinito è False
.
Le versioni di Python precedenti alla 3.6 non garantivano l'ordine delle chiavi nei dizionari. Per garantire che l'ordine delle colonne venga mantenuto per le versioni precedenti di Python e Pandas, puoi specificare index=columns
:
>>> df = pd.DataFrame(data=data, index=columns).T
Ora che hai preparato i tuoi dati, sei pronto per iniziare a lavorare con i file!
Utilizzo dei Panda read_csv()
e .to_csv()
Funzioni
Un file con valori separati da virgole (CSV) è un file di testo normale con un .csv
estensione che contiene dati tabulari. Questo è uno dei formati di file più popolari per l'archiviazione di grandi quantità di dati. Ogni riga del file CSV rappresenta una singola riga della tabella. I valori nella stessa riga sono per impostazione predefinita separati da virgole, ma puoi cambiare il separatore in un punto e virgola, tabulazione, spazio o qualche altro carattere.
Scrivi un file CSV
Puoi salvare i tuoi Panda DataFrame
come file CSV con .to_csv()
:
>>> df.to_csv('data.csv')
Questo è tutto! Hai creato il file data.csv
nella directory di lavoro corrente. Puoi espandere il blocco di codice qui sotto per vedere come dovrebbe apparire il tuo file CSV:
,COUNTRY,POP,AREA,GDP,CONT,IND_DAY
CHN,China,1398.72,9596.96,12234.78,Asia,
IND,India,1351.16,3287.26,2575.67,Asia,1947-08-15
USA,US,329.74,9833.52,19485.39,N.America,1776-07-04
IDN,Indonesia,268.07,1910.93,1015.54,Asia,1945-08-17
BRA,Brazil,210.32,8515.77,2055.51,S.America,1822-09-07
PAK,Pakistan,205.71,881.91,302.14,Asia,1947-08-14
NGA,Nigeria,200.96,923.77,375.77,Africa,1960-10-01
BGD,Bangladesh,167.09,147.57,245.63,Asia,1971-03-26
RUS,Russia,146.79,17098.25,1530.75,,1992-06-12
MEX,Mexico,126.58,1964.38,1158.23,N.America,1810-09-16
JPN,Japan,126.22,377.97,4872.42,Asia,
DEU,Germany,83.02,357.11,3693.2,Europe,
FRA,France,67.02,640.68,2582.49,Europe,1789-07-14
GBR,UK,66.44,242.5,2631.23,Europe,
ITA,Italy,60.36,301.34,1943.84,Europe,
ARG,Argentina,44.94,2780.4,637.49,S.America,1816-07-09
DZA,Algeria,43.38,2381.74,167.56,Africa,1962-07-05
CAN,Canada,37.59,9984.67,1647.12,N.America,1867-07-01
AUS,Australia,25.47,7692.02,1408.68,Oceania,
KAZ,Kazakhstan,18.53,2724.9,159.41,Asia,1991-12-16
Questo file di testo contiene i dati separati da virgole . La prima colonna contiene le etichette di riga. In alcuni casi, li troverai irrilevanti. Se non vuoi mantenerli, puoi passare l'argomento index=False
a .to_csv()
.
Leggi un file CSV
Una volta che i tuoi dati sono stati salvati in un file CSV, probabilmente vorrai caricarli e utilizzarli di tanto in tanto. Puoi farlo con Pandas read_csv()
funzione:
>>> df = pd.read_csv('data.csv', index_col=0)
>>> df
COUNTRY POP AREA GDP CONT IND_DAY
CHN China 1398.72 9596.96 12234.78 Asia NaN
IND India 1351.16 3287.26 2575.67 Asia 1947-08-15
USA US 329.74 9833.52 19485.39 N.America 1776-07-04
IDN Indonesia 268.07 1910.93 1015.54 Asia 1945-08-17
BRA Brazil 210.32 8515.77 2055.51 S.America 1822-09-07
PAK Pakistan 205.71 881.91 302.14 Asia 1947-08-14
NGA Nigeria 200.96 923.77 375.77 Africa 1960-10-01
BGD Bangladesh 167.09 147.57 245.63 Asia 1971-03-26
RUS Russia 146.79 17098.25 1530.75 NaN 1992-06-12
MEX Mexico 126.58 1964.38 1158.23 N.America 1810-09-16
JPN Japan 126.22 377.97 4872.42 Asia NaN
DEU Germany 83.02 357.11 3693.20 Europe NaN
FRA France 67.02 640.68 2582.49 Europe 1789-07-14
GBR UK 66.44 242.50 2631.23 Europe NaN
ITA Italy 60.36 301.34 1943.84 Europe NaN
ARG Argentina 44.94 2780.40 637.49 S.America 1816-07-09
DZA Algeria 43.38 2381.74 167.56 Africa 1962-07-05
CAN Canada 37.59 9984.67 1647.12 N.America 1867-07-01
AUS Australia 25.47 7692.02 1408.68 Oceania NaN
KAZ Kazakhstan 18.53 2724.90 159.41 Asia 1991-12-16
In questo caso, i Panda read_csv()
la funzione restituisce un nuovo DataFrame
con i dati e le etichette del file data.csv
, che hai specificato con il primo argomento. Questa stringa può essere qualsiasi percorso valido, inclusi gli URL.
Il parametro index_col
specifica la colonna del file CSV che contiene le etichette di riga. Assegnare un indice di colonna in base zero a questo parametro. Dovresti determinare il valore di index_col
quando il file CSV contiene le etichette di riga per evitare di caricarle come dati.
Imparerai di più sull'utilizzo di Panda con file CSV più avanti in questo tutorial. Puoi anche controllare Lettura e scrittura di file CSV in Python per vedere come gestire i file CSV anche con la libreria Python integrata csv.
Utilizzo di Panda per scrivere e leggere file Excel
Microsoft Excel è probabilmente il software per fogli di calcolo più utilizzato. Mentre le versioni precedenti utilizzavano .xls
binario file, Excel 2007 ha introdotto il nuovo .xlsx
basato su XML file. Puoi leggere e scrivere file Excel in Panda, in modo simile ai file CSV. Tuttavia, dovrai prima installare i seguenti pacchetti Python:
- xlwt per scrivere in
.xls
file - openpyxl o XlsxWriter per scrivere su
.xlsx
file - xlrd per leggere i file Excel
Puoi installarli usando pip con un solo comando:
$ pip install xlwt openpyxl xlsxwriter xlrd
Puoi anche usare Conda:
$ conda install xlwt openpyxl xlsxwriter xlrd
Tieni presente che non è necessario installare tutto questi pacchetti. Ad esempio, non hai bisogno sia di openpyxl che di XlsxWriter. Se lavorerai solo con .xls
file, quindi non ne hai bisogno! Tuttavia, se intendi lavorare solo con .xlsx
file, allora avrai bisogno di almeno uno di essi, ma non di xlwt
. Prenditi del tempo per decidere quali pacchetti sono adatti al tuo progetto.
Scrivi un file Excel
Una volta installati questi pacchetti, puoi salvare il tuo DataFrame
in un file Excel con .to_excel()
:
>>> df.to_excel('data.xlsx')
L'argomento 'data.xlsx'
rappresenta il file di destinazione e, facoltativamente, il suo percorso. L'istruzione precedente dovrebbe creare il file data.xlsx
nella directory di lavoro corrente. Quel file dovrebbe assomigliare a questo:
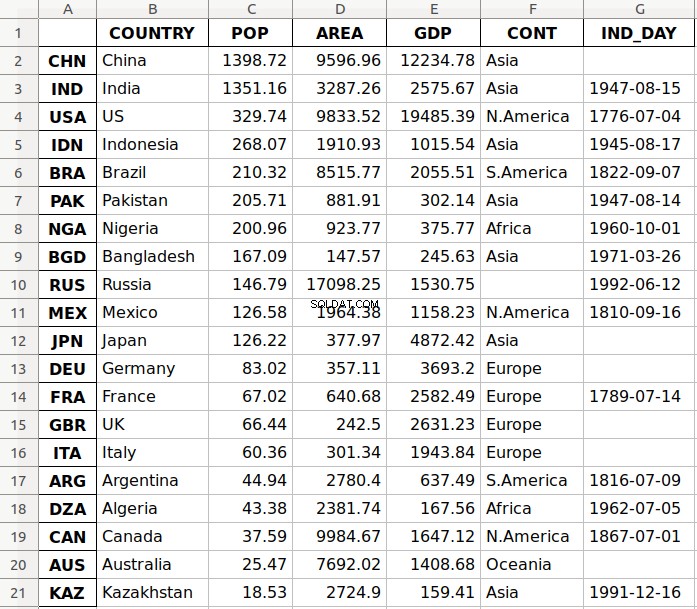
La prima colonna del file contiene le etichette delle righe, mentre le altre colonne memorizzano i dati.
Leggi un file Excel
Puoi caricare dati da file Excel con read_excel()
:
>>> df = pd.read_excel('data.xlsx', index_col=0)
>>> df
COUNTRY POP AREA GDP CONT IND_DAY
CHN China 1398.72 9596.96 12234.78 Asia NaN
IND India 1351.16 3287.26 2575.67 Asia 1947-08-15
USA US 329.74 9833.52 19485.39 N.America 1776-07-04
IDN Indonesia 268.07 1910.93 1015.54 Asia 1945-08-17
BRA Brazil 210.32 8515.77 2055.51 S.America 1822-09-07
PAK Pakistan 205.71 881.91 302.14 Asia 1947-08-14
NGA Nigeria 200.96 923.77 375.77 Africa 1960-10-01
BGD Bangladesh 167.09 147.57 245.63 Asia 1971-03-26
RUS Russia 146.79 17098.25 1530.75 NaN 1992-06-12
MEX Mexico 126.58 1964.38 1158.23 N.America 1810-09-16
JPN Japan 126.22 377.97 4872.42 Asia NaN
DEU Germany 83.02 357.11 3693.20 Europe NaN
FRA France 67.02 640.68 2582.49 Europe 1789-07-14
GBR UK 66.44 242.50 2631.23 Europe NaN
ITA Italy 60.36 301.34 1943.84 Europe NaN
ARG Argentina 44.94 2780.40 637.49 S.America 1816-07-09
DZA Algeria 43.38 2381.74 167.56 Africa 1962-07-05
CAN Canada 37.59 9984.67 1647.12 N.America 1867-07-01
AUS Australia 25.47 7692.02 1408.68 Oceania NaN
KAZ Kazakhstan 18.53 2724.90 159.41 Asia 1991-12-16
read_excel()
restituisce un nuovo DataFrame
che contiene i valori di data.xlsx
. Puoi anche usare read_excel()
con fogli di lavoro OpenDocument o .ods
file.
Imparerai di più sull'utilizzo dei file Excel più avanti in questo tutorial. Puoi anche controllare Utilizzo di Panda per leggere file Excel di grandi dimensioni in Python.
Comprensione dell'API Pandas IO
Strumenti Panda IO è l'API che ti permette di salvare i contenuti di Series
e DataFrame
oggetti negli appunti, oggetti o file di vario tipo. Consente inoltre di caricare dati dagli appunti, oggetti o file.
Scrivi file
Series
e DataFrame
gli oggetti dispongono di metodi che consentono di scrivere dati ed etichette negli appunti o nei file. Sono denominati con il modello .to_<file-type>()
, dove <file-type>
è il tipo del file di destinazione.
Hai imparato a conoscere .to_csv()
e .to_excel()
, ma ce ne sono altri, tra cui:
.to_json()
.to_html()
.to_sql()
.to_pickle()
Ci sono ancora più tipi di file in cui puoi scrivere, quindi questo elenco non è esaustivo.
Nota: Per trovare metodi simili, controlla la documentazione ufficiale su serializzazione, IO e conversione relativa a Series
e DataFrame
oggetti.
Questi metodi hanno parametri che specificano il percorso del file di destinazione in cui sono stati salvati i dati e le etichette. Questo è obbligatorio in alcuni casi e facoltativo in altri. Se questa opzione è disponibile e scegli di ometterla, i metodi restituiscono gli oggetti (come stringhe o iterabili) con il contenuto di DataFrame
istanze.
Il parametro facoltativo compression
decide come comprimere il file con i dati e le etichette. Imparerai di più in seguito. Ci sono alcuni altri parametri, ma sono per lo più specifici di uno o più metodi. Non li approfondirai in dettaglio qui.
Leggi file
Le funzioni Panda per la lettura del contenuto dei file sono denominate usando il modello .read_<file-type>()
, dove <file-type>
indica il tipo di file da leggere. Hai già visto i Panda read_csv()
e read_excel()
funzioni. Eccone altri:
read_json()
read_html()
read_sql()
read_pickle()
Queste funzioni hanno un parametro che specifica il percorso del file di destinazione. Può essere qualsiasi stringa valida che rappresenta il percorso, su una macchina locale o in un URL. Sono accettabili anche altri oggetti a seconda del tipo di file.
Il parametro facoltativo compression
determina il tipo di decompressione da utilizzare per i file compressi. Lo imparerai più avanti in questo tutorial. Esistono altri parametri, ma sono specifici di una o più funzioni. Non li approfondirai in dettaglio qui.
Lavorare con diversi tipi di file
La libreria Pandas offre un'ampia gamma di possibilità per salvare i dati su file e caricare dati da file. In questa sezione imparerai di più su come lavorare con i file CSV ed Excel. Vedrai anche come utilizzare altri tipi di file, come JSON, pagine Web, database e file pickle Python.
File CSV
Hai già imparato a leggere e scrivere file CSV. Ora scaviamo un po' più a fondo nei dettagli. Quando usi .to_csv()
per salvare il tuo DataFrame
, puoi fornire un argomento per il parametro path_or_buf
per specificare il percorso, il nome e l'estensione del file di destinazione.
path_or_buf
è il primo argomento .to_csv()
otterrà. Può essere qualsiasi stringa che rappresenta un percorso file valido che include il nome del file e la sua estensione. L'hai visto in un esempio precedente. Tuttavia, se ometti path_or_buf
, quindi .to_csv()
non creerà alcun file. Invece, restituirà la stringa corrispondente:
>>> df = pd.DataFrame(data=data).T
>>> s = df.to_csv()
>>> print(s)
,COUNTRY,POP,AREA,GDP,CONT,IND_DAY
CHN,China,1398.72,9596.96,12234.78,Asia,
IND,India,1351.16,3287.26,2575.67,Asia,1947-08-15
USA,US,329.74,9833.52,19485.39,N.America,1776-07-04
IDN,Indonesia,268.07,1910.93,1015.54,Asia,1945-08-17
BRA,Brazil,210.32,8515.77,2055.51,S.America,1822-09-07
PAK,Pakistan,205.71,881.91,302.14,Asia,1947-08-14
NGA,Nigeria,200.96,923.77,375.77,Africa,1960-10-01
BGD,Bangladesh,167.09,147.57,245.63,Asia,1971-03-26
RUS,Russia,146.79,17098.25,1530.75,,1992-06-12
MEX,Mexico,126.58,1964.38,1158.23,N.America,1810-09-16
JPN,Japan,126.22,377.97,4872.42,Asia,
DEU,Germany,83.02,357.11,3693.2,Europe,
FRA,France,67.02,640.68,2582.49,Europe,1789-07-14
GBR,UK,66.44,242.5,2631.23,Europe,
ITA,Italy,60.36,301.34,1943.84,Europe,
ARG,Argentina,44.94,2780.4,637.49,S.America,1816-07-09
DZA,Algeria,43.38,2381.74,167.56,Africa,1962-07-05
CAN,Canada,37.59,9984.67,1647.12,N.America,1867-07-01
AUS,Australia,25.47,7692.02,1408.68,Oceania,
KAZ,Kazakhstan,18.53,2724.9,159.41,Asia,1991-12-16
Ora hai la stringa s
invece di un file CSV. Hai anche alcuni valori mancanti nel tuo DataFrame
oggetto. Ad esempio, il continente per la Russia ei giorni dell'indipendenza per diversi paesi (Cina, Giappone e così via) non sono disponibili. Nella scienza dei dati e nell'apprendimento automatico, è necessario gestire con attenzione i valori mancanti. Panda eccelle qui! Per impostazione predefinita, Pandas utilizza il valore NaN per sostituire i valori mancanti.
Nota: nan
, che sta per "non un numero", è un particolare valore a virgola mobile in Python.
Puoi ottenere un nan
valore con una delle seguenti funzioni:
float('nan')
math.nan
numpy.nan
Il continente che corrisponde alla Russia in df
è nan
:
>>> df.loc['RUS', 'CONT']
nan
Questo esempio utilizza .loc[]
per ottenere dati con i nomi di riga e colonna specificati.
Quando salvi il tuo DataFrame
in un file CSV, stringhe vuote (''
) rappresenterà i dati mancanti. Puoi vederlo entrambi nel tuo file data.csv
e nella stringa s
. Se vuoi modificare questo comportamento, usa il parametro facoltativo na_rep
:
>>> df.to_csv('new-data.csv', na_rep='(missing)')
Questo codice produce il file new-data.csv
dove i valori mancanti non sono più stringhe vuote. Puoi espandere il blocco di codice qui sotto per vedere come dovrebbe apparire questo file:
,COUNTRY,POP,AREA,GDP,CONT,IND_DAY
CHN,China,1398.72,9596.96,12234.78,Asia,(missing)
IND,India,1351.16,3287.26,2575.67,Asia,1947-08-15
USA,US,329.74,9833.52,19485.39,N.America,1776-07-04
IDN,Indonesia,268.07,1910.93,1015.54,Asia,1945-08-17
BRA,Brazil,210.32,8515.77,2055.51,S.America,1822-09-07
PAK,Pakistan,205.71,881.91,302.14,Asia,1947-08-14
NGA,Nigeria,200.96,923.77,375.77,Africa,1960-10-01
BGD,Bangladesh,167.09,147.57,245.63,Asia,1971-03-26
RUS,Russia,146.79,17098.25,1530.75,(missing),1992-06-12
MEX,Mexico,126.58,1964.38,1158.23,N.America,1810-09-16
JPN,Japan,126.22,377.97,4872.42,Asia,(missing)
DEU,Germany,83.02,357.11,3693.2,Europe,(missing)
FRA,France,67.02,640.68,2582.49,Europe,1789-07-14
GBR,UK,66.44,242.5,2631.23,Europe,(missing)
ITA,Italy,60.36,301.34,1943.84,Europe,(missing)
ARG,Argentina,44.94,2780.4,637.49,S.America,1816-07-09
DZA,Algeria,43.38,2381.74,167.56,Africa,1962-07-05
CAN,Canada,37.59,9984.67,1647.12,N.America,1867-07-01
AUS,Australia,25.47,7692.02,1408.68,Oceania,(missing)
KAZ,Kazakhstan,18.53,2724.9,159.41,Asia,1991-12-16
Now, the string '(missing)'
in the file corresponds to the nan
values from df
.
When Pandas reads files, it considers the empty string (''
) and a few others as missing values by default:
'nan'
'-nan'
'NA'
'N/A'
'NaN'
'null'
If you don’t want this behavior, then you can pass keep_default_na=False
to the Pandas read_csv()
funzione. To specify other labels for missing values, use the parameter na_values
:
>>> pd.read_csv('new-data.csv', index_col=0, na_values='(missing)')
COUNTRY POP AREA GDP CONT IND_DAY
CHN China 1398.72 9596.96 12234.78 Asia NaN
IND India 1351.16 3287.26 2575.67 Asia 1947-08-15
USA US 329.74 9833.52 19485.39 N.America 1776-07-04
IDN Indonesia 268.07 1910.93 1015.54 Asia 1945-08-17
BRA Brazil 210.32 8515.77 2055.51 S.America 1822-09-07
PAK Pakistan 205.71 881.91 302.14 Asia 1947-08-14
NGA Nigeria 200.96 923.77 375.77 Africa 1960-10-01
BGD Bangladesh 167.09 147.57 245.63 Asia 1971-03-26
RUS Russia 146.79 17098.25 1530.75 NaN 1992-06-12
MEX Mexico 126.58 1964.38 1158.23 N.America 1810-09-16
JPN Japan 126.22 377.97 4872.42 Asia NaN
DEU Germany 83.02 357.11 3693.20 Europe NaN
FRA France 67.02 640.68 2582.49 Europe 1789-07-14
GBR UK 66.44 242.50 2631.23 Europe NaN
ITA Italy 60.36 301.34 1943.84 Europe NaN
ARG Argentina 44.94 2780.40 637.49 S.America 1816-07-09
DZA Algeria 43.38 2381.74 167.56 Africa 1962-07-05
CAN Canada 37.59 9984.67 1647.12 N.America 1867-07-01
AUS Australia 25.47 7692.02 1408.68 Oceania NaN
KAZ Kazakhstan 18.53 2724.90 159.41 Asia 1991-12-16
Here, you’ve marked the string '(missing)'
as a new missing data label, and Pandas replaced it with nan
when it read the file.
When you load data from a file, Pandas assigns the data types to the values of each column by default. You can check these types with .dtypes
:
>>> df = pd.read_csv('data.csv', index_col=0)
>>> df.dtypes
COUNTRY object
POP float64
AREA float64
GDP float64
CONT object
IND_DAY object
dtype: object
The columns with strings and dates ('COUNTRY'
, 'CONT'
, and 'IND_DAY'
) have the data type object
. Meanwhile, the numeric columns contain 64-bit floating-point numbers (float64
).
You can use the parameter dtype
to specify the desired data types and parse_dates
to force use of datetimes:
>>> dtypes = {'POP': 'float32', 'AREA': 'float32', 'GDP': 'float32'}
>>> df = pd.read_csv('data.csv', index_col=0, dtype=dtypes,
... parse_dates=['IND_DAY'])
>>> df.dtypes
COUNTRY object
POP float32
AREA float32
GDP float32
CONT object
IND_DAY datetime64[ns]
dtype: object
>>> df['IND_DAY']
CHN NaT
IND 1947-08-15
USA 1776-07-04
IDN 1945-08-17
BRA 1822-09-07
PAK 1947-08-14
NGA 1960-10-01
BGD 1971-03-26
RUS 1992-06-12
MEX 1810-09-16
JPN NaT
DEU NaT
FRA 1789-07-14
GBR NaT
ITA NaT
ARG 1816-07-09
DZA 1962-07-05
CAN 1867-07-01
AUS NaT
KAZ 1991-12-16
Name: IND_DAY, dtype: datetime64[ns]
Now, you have 32-bit floating-point numbers (float32
) as specified with dtype
. These differ slightly from the original 64-bit numbers because of smaller precision . The values in the last column are considered as dates and have the data type datetime64
. That’s why the NaN
values in this column are replaced with NaT
.
Now that you have real dates, you can save them in the format you like:
>>>>>> df = pd.read_csv('data.csv', index_col=0, parse_dates=['IND_DAY'])
>>> df.to_csv('formatted-data.csv', date_format='%B %d, %Y')
Here, you’ve specified the parameter date_format
to be '%B %d, %Y'
. You can expand the code block below to see the resulting file:
,COUNTRY,POP,AREA,GDP,CONT,IND_DAY
CHN,China,1398.72,9596.96,12234.78,Asia,
IND,India,1351.16,3287.26,2575.67,Asia,"August 15, 1947"
USA,US,329.74,9833.52,19485.39,N.America,"July 04, 1776"
IDN,Indonesia,268.07,1910.93,1015.54,Asia,"August 17, 1945"
BRA,Brazil,210.32,8515.77,2055.51,S.America,"September 07, 1822"
PAK,Pakistan,205.71,881.91,302.14,Asia,"August 14, 1947"
NGA,Nigeria,200.96,923.77,375.77,Africa,"October 01, 1960"
BGD,Bangladesh,167.09,147.57,245.63,Asia,"March 26, 1971"
RUS,Russia,146.79,17098.25,1530.75,,"June 12, 1992"
MEX,Mexico,126.58,1964.38,1158.23,N.America,"September 16, 1810"
JPN,Japan,126.22,377.97,4872.42,Asia,
DEU,Germany,83.02,357.11,3693.2,Europe,
FRA,France,67.02,640.68,2582.49,Europe,"July 14, 1789"
GBR,UK,66.44,242.5,2631.23,Europe,
ITA,Italy,60.36,301.34,1943.84,Europe,
ARG,Argentina,44.94,2780.4,637.49,S.America,"July 09, 1816"
DZA,Algeria,43.38,2381.74,167.56,Africa,"July 05, 1962"
CAN,Canada,37.59,9984.67,1647.12,N.America,"July 01, 1867"
AUS,Australia,25.47,7692.02,1408.68,Oceania,
KAZ,Kazakhstan,18.53,2724.9,159.41,Asia,"December 16, 1991"
The format of the dates is different now. The format '%B %d, %Y'
means the date will first display the full name of the month, then the day followed by a comma, and finally the full year.
There are several other optional parameters that you can use with .to_csv()
:
sep
denotes a values separator.decimal
indicates a decimal separator.encoding
sets the file encoding.header
specifies whether you want to write column labels in the file.
Here’s how you would pass arguments for sep
and header
:
>>> s = df.to_csv(sep=';', header=False)
>>> print(s)
CHN;China;1398.72;9596.96;12234.78;Asia;
IND;India;1351.16;3287.26;2575.67;Asia;1947-08-15
USA;US;329.74;9833.52;19485.39;N.America;1776-07-04
IDN;Indonesia;268.07;1910.93;1015.54;Asia;1945-08-17
BRA;Brazil;210.32;8515.77;2055.51;S.America;1822-09-07
PAK;Pakistan;205.71;881.91;302.14;Asia;1947-08-14
NGA;Nigeria;200.96;923.77;375.77;Africa;1960-10-01
BGD;Bangladesh;167.09;147.57;245.63;Asia;1971-03-26
RUS;Russia;146.79;17098.25;1530.75;;1992-06-12
MEX;Mexico;126.58;1964.38;1158.23;N.America;1810-09-16
JPN;Japan;126.22;377.97;4872.42;Asia;
DEU;Germany;83.02;357.11;3693.2;Europe;
FRA;France;67.02;640.68;2582.49;Europe;1789-07-14
GBR;UK;66.44;242.5;2631.23;Europe;
ITA;Italy;60.36;301.34;1943.84;Europe;
ARG;Argentina;44.94;2780.4;637.49;S.America;1816-07-09
DZA;Algeria;43.38;2381.74;167.56;Africa;1962-07-05
CAN;Canada;37.59;9984.67;1647.12;N.America;1867-07-01
AUS;Australia;25.47;7692.02;1408.68;Oceania;
KAZ;Kazakhstan;18.53;2724.9;159.41;Asia;1991-12-16
The data is separated with a semicolon (';'
) because you’ve specified sep=';'
. Also, since you passed header=False
, you see your data without the header row of column names.
The Pandas read_csv()
function has many additional options for managing missing data, working with dates and times, quoting, encoding, handling errors, and more. For instance, if you have a file with one data column and want to get a Series
object instead of a DataFrame
, then you can pass squeeze=True
to read_csv()
. You’ll learn later on about data compression and decompression, as well as how to skip rows and columns.
JSON Files
JSON stands for JavaScript object notation. JSON files are plaintext files used for data interchange, and humans can read them easily. They follow the ISO/IEC 21778:2017 and ECMA-404 standards and use the .json
estensione. Python and Pandas work well with JSON files, as Python’s json library offers built-in support for them.
You can save the data from your DataFrame
to a JSON file with .to_json()
. Start by creating a DataFrame
object again. Use the dictionary data
that holds the data about countries and then apply .to_json()
:
>>> df = pd.DataFrame(data=data).T
>>> df.to_json('data-columns.json')
This code produces the file data-columns.json
. You can expand the code block below to see how this file should look:
{"COUNTRY":{"CHN":"China","IND":"India","USA":"US","IDN":"Indonesia","BRA":"Brazil","PAK":"Pakistan","NGA":"Nigeria","BGD":"Bangladesh","RUS":"Russia","MEX":"Mexico","JPN":"Japan","DEU":"Germany","FRA":"France","GBR":"UK","ITA":"Italy","ARG":"Argentina","DZA":"Algeria","CAN":"Canada","AUS":"Australia","KAZ":"Kazakhstan"},"POP":{"CHN":1398.72,"IND":1351.16,"USA":329.74,"IDN":268.07,"BRA":210.32,"PAK":205.71,"NGA":200.96,"BGD":167.09,"RUS":146.79,"MEX":126.58,"JPN":126.22,"DEU":83.02,"FRA":67.02,"GBR":66.44,"ITA":60.36,"ARG":44.94,"DZA":43.38,"CAN":37.59,"AUS":25.47,"KAZ":18.53},"AREA":{"CHN":9596.96,"IND":3287.26,"USA":9833.52,"IDN":1910.93,"BRA":8515.77,"PAK":881.91,"NGA":923.77,"BGD":147.57,"RUS":17098.25,"MEX":1964.38,"JPN":377.97,"DEU":357.11,"FRA":640.68,"GBR":242.5,"ITA":301.34,"ARG":2780.4,"DZA":2381.74,"CAN":9984.67,"AUS":7692.02,"KAZ":2724.9},"GDP":{"CHN":12234.78,"IND":2575.67,"USA":19485.39,"IDN":1015.54,"BRA":2055.51,"PAK":302.14,"NGA":375.77,"BGD":245.63,"RUS":1530.75,"MEX":1158.23,"JPN":4872.42,"DEU":3693.2,"FRA":2582.49,"GBR":2631.23,"ITA":1943.84,"ARG":637.49,"DZA":167.56,"CAN":1647.12,"AUS":1408.68,"KAZ":159.41},"CONT":{"CHN":"Asia","IND":"Asia","USA":"N.America","IDN":"Asia","BRA":"S.America","PAK":"Asia","NGA":"Africa","BGD":"Asia","RUS":null,"MEX":"N.America","JPN":"Asia","DEU":"Europe","FRA":"Europe","GBR":"Europe","ITA":"Europe","ARG":"S.America","DZA":"Africa","CAN":"N.America","AUS":"Oceania","KAZ":"Asia"},"IND_DAY":{"CHN":null,"IND":"1947-08-15","USA":"1776-07-04","IDN":"1945-08-17","BRA":"1822-09-07","PAK":"1947-08-14","NGA":"1960-10-01","BGD":"1971-03-26","RUS":"1992-06-12","MEX":"1810-09-16","JPN":null,"DEU":null,"FRA":"1789-07-14","GBR":null,"ITA":null,"ARG":"1816-07-09","DZA":"1962-07-05","CAN":"1867-07-01","AUS":null,"KAZ":"1991-12-16"}}
data-columns.json
has one large dictionary with the column labels as keys and the corresponding inner dictionaries as values.
You can get a different file structure if you pass an argument for the optional parameter orient
:
>>> df.to_json('data-index.json', orient='index')
The orient
parameter defaults to 'columns'
. Here, you’ve set it to index
.
You should get a new file data-index.json
. You can expand the code block below to see the changes:
{"CHN":{"COUNTRY":"China","POP":1398.72,"AREA":9596.96,"GDP":12234.78,"CONT":"Asia","IND_DAY":null},"IND":{"COUNTRY":"India","POP":1351.16,"AREA":3287.26,"GDP":2575.67,"CONT":"Asia","IND_DAY":"1947-08-15"},"USA":{"COUNTRY":"US","POP":329.74,"AREA":9833.52,"GDP":19485.39,"CONT":"N.America","IND_DAY":"1776-07-04"},"IDN":{"COUNTRY":"Indonesia","POP":268.07,"AREA":1910.93,"GDP":1015.54,"CONT":"Asia","IND_DAY":"1945-08-17"},"BRA":{"COUNTRY":"Brazil","POP":210.32,"AREA":8515.77,"GDP":2055.51,"CONT":"S.America","IND_DAY":"1822-09-07"},"PAK":{"COUNTRY":"Pakistan","POP":205.71,"AREA":881.91,"GDP":302.14,"CONT":"Asia","IND_DAY":"1947-08-14"},"NGA":{"COUNTRY":"Nigeria","POP":200.96,"AREA":923.77,"GDP":375.77,"CONT":"Africa","IND_DAY":"1960-10-01"},"BGD":{"COUNTRY":"Bangladesh","POP":167.09,"AREA":147.57,"GDP":245.63,"CONT":"Asia","IND_DAY":"1971-03-26"},"RUS":{"COUNTRY":"Russia","POP":146.79,"AREA":17098.25,"GDP":1530.75,"CONT":null,"IND_DAY":"1992-06-12"},"MEX":{"COUNTRY":"Mexico","POP":126.58,"AREA":1964.38,"GDP":1158.23,"CONT":"N.America","IND_DAY":"1810-09-16"},"JPN":{"COUNTRY":"Japan","POP":126.22,"AREA":377.97,"GDP":4872.42,"CONT":"Asia","IND_DAY":null},"DEU":{"COUNTRY":"Germany","POP":83.02,"AREA":357.11,"GDP":3693.2,"CONT":"Europe","IND_DAY":null},"FRA":{"COUNTRY":"France","POP":67.02,"AREA":640.68,"GDP":2582.49,"CONT":"Europe","IND_DAY":"1789-07-14"},"GBR":{"COUNTRY":"UK","POP":66.44,"AREA":242.5,"GDP":2631.23,"CONT":"Europe","IND_DAY":null},"ITA":{"COUNTRY":"Italy","POP":60.36,"AREA":301.34,"GDP":1943.84,"CONT":"Europe","IND_DAY":null},"ARG":{"COUNTRY":"Argentina","POP":44.94,"AREA":2780.4,"GDP":637.49,"CONT":"S.America","IND_DAY":"1816-07-09"},"DZA":{"COUNTRY":"Algeria","POP":43.38,"AREA":2381.74,"GDP":167.56,"CONT":"Africa","IND_DAY":"1962-07-05"},"CAN":{"COUNTRY":"Canada","POP":37.59,"AREA":9984.67,"GDP":1647.12,"CONT":"N.America","IND_DAY":"1867-07-01"},"AUS":{"COUNTRY":"Australia","POP":25.47,"AREA":7692.02,"GDP":1408.68,"CONT":"Oceania","IND_DAY":null},"KAZ":{"COUNTRY":"Kazakhstan","POP":18.53,"AREA":2724.9,"GDP":159.41,"CONT":"Asia","IND_DAY":"1991-12-16"}}
data-index.json
also has one large dictionary, but this time the row labels are the keys, and the inner dictionaries are the values.
There are few more options for orient
. One of them is 'records'
:
>>> df.to_json('data-records.json', orient='records')
This code should yield the file data-records.json
. You can expand the code block below to see the content:
[{"COUNTRY":"China","POP":1398.72,"AREA":9596.96,"GDP":12234.78,"CONT":"Asia","IND_DAY":null},{"COUNTRY":"India","POP":1351.16,"AREA":3287.26,"GDP":2575.67,"CONT":"Asia","IND_DAY":"1947-08-15"},{"COUNTRY":"US","POP":329.74,"AREA":9833.52,"GDP":19485.39,"CONT":"N.America","IND_DAY":"1776-07-04"},{"COUNTRY":"Indonesia","POP":268.07,"AREA":1910.93,"GDP":1015.54,"CONT":"Asia","IND_DAY":"1945-08-17"},{"COUNTRY":"Brazil","POP":210.32,"AREA":8515.77,"GDP":2055.51,"CONT":"S.America","IND_DAY":"1822-09-07"},{"COUNTRY":"Pakistan","POP":205.71,"AREA":881.91,"GDP":302.14,"CONT":"Asia","IND_DAY":"1947-08-14"},{"COUNTRY":"Nigeria","POP":200.96,"AREA":923.77,"GDP":375.77,"CONT":"Africa","IND_DAY":"1960-10-01"},{"COUNTRY":"Bangladesh","POP":167.09,"AREA":147.57,"GDP":245.63,"CONT":"Asia","IND_DAY":"1971-03-26"},{"COUNTRY":"Russia","POP":146.79,"AREA":17098.25,"GDP":1530.75,"CONT":null,"IND_DAY":"1992-06-12"},{"COUNTRY":"Mexico","POP":126.58,"AREA":1964.38,"GDP":1158.23,"CONT":"N.America","IND_DAY":"1810-09-16"},{"COUNTRY":"Japan","POP":126.22,"AREA":377.97,"GDP":4872.42,"CONT":"Asia","IND_DAY":null},{"COUNTRY":"Germany","POP":83.02,"AREA":357.11,"GDP":3693.2,"CONT":"Europe","IND_DAY":null},{"COUNTRY":"France","POP":67.02,"AREA":640.68,"GDP":2582.49,"CONT":"Europe","IND_DAY":"1789-07-14"},{"COUNTRY":"UK","POP":66.44,"AREA":242.5,"GDP":2631.23,"CONT":"Europe","IND_DAY":null},{"COUNTRY":"Italy","POP":60.36,"AREA":301.34,"GDP":1943.84,"CONT":"Europe","IND_DAY":null},{"COUNTRY":"Argentina","POP":44.94,"AREA":2780.4,"GDP":637.49,"CONT":"S.America","IND_DAY":"1816-07-09"},{"COUNTRY":"Algeria","POP":43.38,"AREA":2381.74,"GDP":167.56,"CONT":"Africa","IND_DAY":"1962-07-05"},{"COUNTRY":"Canada","POP":37.59,"AREA":9984.67,"GDP":1647.12,"CONT":"N.America","IND_DAY":"1867-07-01"},{"COUNTRY":"Australia","POP":25.47,"AREA":7692.02,"GDP":1408.68,"CONT":"Oceania","IND_DAY":null},{"COUNTRY":"Kazakhstan","POP":18.53,"AREA":2724.9,"GDP":159.41,"CONT":"Asia","IND_DAY":"1991-12-16"}]
data-records.json
holds a list with one dictionary for each row. The row labels are not written.
You can get another interesting file structure with orient='split'
:
>>> df.to_json('data-split.json', orient='split')
The resulting file is data-split.json
. You can expand the code block below to see how this file should look:
{"columns":["COUNTRY","POP","AREA","GDP","CONT","IND_DAY"],"index":["CHN","IND","USA","IDN","BRA","PAK","NGA","BGD","RUS","MEX","JPN","DEU","FRA","GBR","ITA","ARG","DZA","CAN","AUS","KAZ"],"data":[["China",1398.72,9596.96,12234.78,"Asia",null],["India",1351.16,3287.26,2575.67,"Asia","1947-08-15"],["US",329.74,9833.52,19485.39,"N.America","1776-07-04"],["Indonesia",268.07,1910.93,1015.54,"Asia","1945-08-17"],["Brazil",210.32,8515.77,2055.51,"S.America","1822-09-07"],["Pakistan",205.71,881.91,302.14,"Asia","1947-08-14"],["Nigeria",200.96,923.77,375.77,"Africa","1960-10-01"],["Bangladesh",167.09,147.57,245.63,"Asia","1971-03-26"],["Russia",146.79,17098.25,1530.75,null,"1992-06-12"],["Mexico",126.58,1964.38,1158.23,"N.America","1810-09-16"],["Japan",126.22,377.97,4872.42,"Asia",null],["Germany",83.02,357.11,3693.2,"Europe",null],["France",67.02,640.68,2582.49,"Europe","1789-07-14"],["UK",66.44,242.5,2631.23,"Europe",null],["Italy",60.36,301.34,1943.84,"Europe",null],["Argentina",44.94,2780.4,637.49,"S.America","1816-07-09"],["Algeria",43.38,2381.74,167.56,"Africa","1962-07-05"],["Canada",37.59,9984.67,1647.12,"N.America","1867-07-01"],["Australia",25.47,7692.02,1408.68,"Oceania",null],["Kazakhstan",18.53,2724.9,159.41,"Asia","1991-12-16"]]}
data-split.json
contains one dictionary that holds the following lists:
- The names of the columns
- The labels of the rows
- The inner lists (two-dimensional sequence) that hold data values
If you don’t provide the value for the optional parameter path_or_buf
that defines the file path, then .to_json()
will return a JSON string instead of writing the results to a file. This behavior is consistent with .to_csv()
.
There are other optional parameters you can use. For instance, you can set index=False
to forgo saving row labels. You can manipulate precision with double_precision
, and dates with date_format
and date_unit
. These last two parameters are particularly important when you have time series among your data:
>>> df = pd.DataFrame(data=data).T
>>> df['IND_DAY'] = pd.to_datetime(df['IND_DAY'])
>>> df.dtypes
COUNTRY object
POP object
AREA object
GDP object
CONT object
IND_DAY datetime64[ns]
dtype: object
>>> df.to_json('data-time.json')
In this example, you’ve created the DataFrame
from the dictionary data
and used to_datetime()
to convert the values in the last column to datetime64
. You can expand the code block below to see the resulting file:
{"COUNTRY":{"CHN":"China","IND":"India","USA":"US","IDN":"Indonesia","BRA":"Brazil","PAK":"Pakistan","NGA":"Nigeria","BGD":"Bangladesh","RUS":"Russia","MEX":"Mexico","JPN":"Japan","DEU":"Germany","FRA":"France","GBR":"UK","ITA":"Italy","ARG":"Argentina","DZA":"Algeria","CAN":"Canada","AUS":"Australia","KAZ":"Kazakhstan"},"POP":{"CHN":1398.72,"IND":1351.16,"USA":329.74,"IDN":268.07,"BRA":210.32,"PAK":205.71,"NGA":200.96,"BGD":167.09,"RUS":146.79,"MEX":126.58,"JPN":126.22,"DEU":83.02,"FRA":67.02,"GBR":66.44,"ITA":60.36,"ARG":44.94,"DZA":43.38,"CAN":37.59,"AUS":25.47,"KAZ":18.53},"AREA":{"CHN":9596.96,"IND":3287.26,"USA":9833.52,"IDN":1910.93,"BRA":8515.77,"PAK":881.91,"NGA":923.77,"BGD":147.57,"RUS":17098.25,"MEX":1964.38,"JPN":377.97,"DEU":357.11,"FRA":640.68,"GBR":242.5,"ITA":301.34,"ARG":2780.4,"DZA":2381.74,"CAN":9984.67,"AUS":7692.02,"KAZ":2724.9},"GDP":{"CHN":12234.78,"IND":2575.67,"USA":19485.39,"IDN":1015.54,"BRA":2055.51,"PAK":302.14,"NGA":375.77,"BGD":245.63,"RUS":1530.75,"MEX":1158.23,"JPN":4872.42,"DEU":3693.2,"FRA":2582.49,"GBR":2631.23,"ITA":1943.84,"ARG":637.49,"DZA":167.56,"CAN":1647.12,"AUS":1408.68,"KAZ":159.41},"CONT":{"CHN":"Asia","IND":"Asia","USA":"N.America","IDN":"Asia","BRA":"S.America","PAK":"Asia","NGA":"Africa","BGD":"Asia","RUS":null,"MEX":"N.America","JPN":"Asia","DEU":"Europe","FRA":"Europe","GBR":"Europe","ITA":"Europe","ARG":"S.America","DZA":"Africa","CAN":"N.America","AUS":"Oceania","KAZ":"Asia"},"IND_DAY":{"CHN":null,"IND":-706320000000,"USA":-6106060800000,"IDN":-769219200000,"BRA":-4648924800000,"PAK":-706406400000,"NGA":-291945600000,"BGD":38793600000,"RUS":708307200000,"MEX":-5026838400000,"JPN":null,"DEU":null,"FRA":-5694969600000,"GBR":null,"ITA":null,"ARG":-4843411200000,"DZA":-236476800000,"CAN":-3234729600000,"AUS":null,"KAZ":692841600000}}
In this file, you have large integers instead of dates for the independence days. That’s because the default value of the optional parameter date_format
is 'epoch'
whenever orient
isn’t 'table'
. This default behavior expresses dates as an epoch in milliseconds relative to midnight on January 1, 1970.
However, if you pass date_format='iso'
, then you’ll get the dates in the ISO 8601 format. In addition, date_unit
decides the units of time:
>>> df = pd.DataFrame(data=data).T
>>> df['IND_DAY'] = pd.to_datetime(df['IND_DAY'])
>>> df.to_json('new-data-time.json', date_format='iso', date_unit='s')
This code produces the following JSON file:
{"COUNTRY":{"CHN":"China","IND":"India","USA":"US","IDN":"Indonesia","BRA":"Brazil","PAK":"Pakistan","NGA":"Nigeria","BGD":"Bangladesh","RUS":"Russia","MEX":"Mexico","JPN":"Japan","DEU":"Germany","FRA":"France","GBR":"UK","ITA":"Italy","ARG":"Argentina","DZA":"Algeria","CAN":"Canada","AUS":"Australia","KAZ":"Kazakhstan"},"POP":{"CHN":1398.72,"IND":1351.16,"USA":329.74,"IDN":268.07,"BRA":210.32,"PAK":205.71,"NGA":200.96,"BGD":167.09,"RUS":146.79,"MEX":126.58,"JPN":126.22,"DEU":83.02,"FRA":67.02,"GBR":66.44,"ITA":60.36,"ARG":44.94,"DZA":43.38,"CAN":37.59,"AUS":25.47,"KAZ":18.53},"AREA":{"CHN":9596.96,"IND":3287.26,"USA":9833.52,"IDN":1910.93,"BRA":8515.77,"PAK":881.91,"NGA":923.77,"BGD":147.57,"RUS":17098.25,"MEX":1964.38,"JPN":377.97,"DEU":357.11,"FRA":640.68,"GBR":242.5,"ITA":301.34,"ARG":2780.4,"DZA":2381.74,"CAN":9984.67,"AUS":7692.02,"KAZ":2724.9},"GDP":{"CHN":12234.78,"IND":2575.67,"USA":19485.39,"IDN":1015.54,"BRA":2055.51,"PAK":302.14,"NGA":375.77,"BGD":245.63,"RUS":1530.75,"MEX":1158.23,"JPN":4872.42,"DEU":3693.2,"FRA":2582.49,"GBR":2631.23,"ITA":1943.84,"ARG":637.49,"DZA":167.56,"CAN":1647.12,"AUS":1408.68,"KAZ":159.41},"CONT":{"CHN":"Asia","IND":"Asia","USA":"N.America","IDN":"Asia","BRA":"S.America","PAK":"Asia","NGA":"Africa","BGD":"Asia","RUS":null,"MEX":"N.America","JPN":"Asia","DEU":"Europe","FRA":"Europe","GBR":"Europe","ITA":"Europe","ARG":"S.America","DZA":"Africa","CAN":"N.America","AUS":"Oceania","KAZ":"Asia"},"IND_DAY":{"CHN":null,"IND":"1947-08-15T00:00:00Z","USA":"1776-07-04T00:00:00Z","IDN":"1945-08-17T00:00:00Z","BRA":"1822-09-07T00:00:00Z","PAK":"1947-08-14T00:00:00Z","NGA":"1960-10-01T00:00:00Z","BGD":"1971-03-26T00:00:00Z","RUS":"1992-06-12T00:00:00Z","MEX":"1810-09-16T00:00:00Z","JPN":null,"DEU":null,"FRA":"1789-07-14T00:00:00Z","GBR":null,"ITA":null,"ARG":"1816-07-09T00:00:00Z","DZA":"1962-07-05T00:00:00Z","CAN":"1867-07-01T00:00:00Z","AUS":null,"KAZ":"1991-12-16T00:00:00Z"}}
The dates in the resulting file are in the ISO 8601 format.
You can load the data from a JSON file with read_json()
:
>>> df = pd.read_json('data-index.json', orient='index',
... convert_dates=['IND_DAY'])
The parameter convert_dates
has a similar purpose as parse_dates
when you use it to read CSV files. The optional parameter orient
is very important because it specifies how Pandas understands the structure of the file.
There are other optional parameters you can use as well:
- Set the encoding with
encoding
. - Manipulate dates with
convert_dates
andkeep_default_dates
. - Impact precision with
dtype
andprecise_float
. - Decode numeric data directly to NumPy arrays with
numpy=True
.
Note that you might lose the order of rows and columns when using the JSON format to store your data.
HTML Files
An HTML is a plaintext file that uses hypertext markup language to help browsers render web pages. The extensions for HTML files are .html
and .htm
. You’ll need to install an HTML parser library like lxml or html5lib to be able to work with HTML files:
$pip install lxml html5lib
You can also use Conda to install the same packages:
$ conda install lxml html5lib
Once you have these libraries, you can save the contents of your DataFrame
as an HTML file with .to_html()
:
df = pd.DataFrame(data=data).T
df.to_html('data.html')
This code generates a file data.html
. You can expand the code block below to see how this file should look:
<table border="1" class="dataframe">
<thead>
<tr style="text-align: right;">
<th></th>
<th>COUNTRY</th>
<th>POP</th>
<th>AREA</th>
<th>GDP</th>
<th>CONT</th>
<th>IND_DAY</th>
</tr>
</thead>
<tbody>
<tr>
<th>CHN</th>
<td>China</td>
<td>1398.72</td>
<td>9596.96</td>
<td>12234.8</td>
<td>Asia</td>
<td>NaN</td>
</tr>
<tr>
<th>IND</th>
<td>India</td>
<td>1351.16</td>
<td>3287.26</td>
<td>2575.67</td>
<td>Asia</td>
<td>1947-08-15</td>
</tr>
<tr>
<th>USA</th>
<td>US</td>
<td>329.74</td>
<td>9833.52</td>
<td>19485.4</td>
<td>N.America</td>
<td>1776-07-04</td>
</tr>
<tr>
<th>IDN</th>
<td>Indonesia</td>
<td>268.07</td>
<td>1910.93</td>
<td>1015.54</td>
<td>Asia</td>
<td>1945-08-17</td>
</tr>
<tr>
<th>BRA</th>
<td>Brazil</td>
<td>210.32</td>
<td>8515.77</td>
<td>2055.51</td>
<td>S.America</td>
<td>1822-09-07</td>
</tr>
<tr>
<th>PAK</th>
<td>Pakistan</td>
<td>205.71</td>
<td>881.91</td>
<td>302.14</td>
<td>Asia</td>
<td>1947-08-14</td>
</tr>
<tr>
<th>NGA</th>
<td>Nigeria</td>
<td>200.96</td>
<td>923.77</td>
<td>375.77</td>
<td>Africa</td>
<td>1960-10-01</td>
</tr>
<tr>
<th>BGD</th>
<td>Bangladesh</td>
<td>167.09</td>
<td>147.57</td>
<td>245.63</td>
<td>Asia</td>
<td>1971-03-26</td>
</tr>
<tr>
<th>RUS</th>
<td>Russia</td>
<td>146.79</td>
<td>17098.2</td>
<td>1530.75</td>
<td>NaN</td>
<td>1992-06-12</td>
</tr>
<tr>
<th>MEX</th>
<td>Mexico</td>
<td>126.58</td>
<td>1964.38</td>
<td>1158.23</td>
<td>N.America</td>
<td>1810-09-16</td>
</tr>
<tr>
<th>JPN</th>
<td>Japan</td>
<td>126.22</td>
<td>377.97</td>
<td>4872.42</td>
<td>Asia</td>
<td>NaN</td>
</tr>
<tr>
<th>DEU</th>
<td>Germany</td>
<td>83.02</td>
<td>357.11</td>
<td>3693.2</td>
<td>Europe</td>
<td>NaN</td>
</tr>
<tr>
<th>FRA</th>
<td>France</td>
<td>67.02</td>
<td>640.68</td>
<td>2582.49</td>
<td>Europe</td>
<td>1789-07-14</td>
</tr>
<tr>
<th>GBR</th>
<td>UK</td>
<td>66.44</td>
<td>242.5</td>
<td>2631.23</td>
<td>Europe</td>
<td>NaN</td>
</tr>
<tr>
<th>ITA</th>
<td>Italy</td>
<td>60.36</td>
<td>301.34</td>
<td>1943.84</td>
<td>Europe</td>
<td>NaN</td>
</tr>
<tr>
<th>ARG</th>
<td>Argentina</td>
<td>44.94</td>
<td>2780.4</td>
<td>637.49</td>
<td>S.America</td>
<td>1816-07-09</td>
</tr>
<tr>
<th>DZA</th>
<td>Algeria</td>
<td>43.38</td>
<td>2381.74</td>
<td>167.56</td>
<td>Africa</td>
<td>1962-07-05</td>
</tr>
<tr>
<th>CAN</th>
<td>Canada</td>
<td>37.59</td>
<td>9984.67</td>
<td>1647.12</td>
<td>N.America</td>
<td>1867-07-01</td>
</tr>
<tr>
<th>AUS</th>
<td>Australia</td>
<td>25.47</td>
<td>7692.02</td>
<td>1408.68</td>
<td>Oceania</td>
<td>NaN</td>
</tr>
<tr>
<th>KAZ</th>
<td>Kazakhstan</td>
<td>18.53</td>
<td>2724.9</td>
<td>159.41</td>
<td>Asia</td>
<td>1991-12-16</td>
</tr>
</tbody>
</table>
This file shows the DataFrame
contents nicely. However, notice that you haven’t obtained an entire web page. You’ve just output the data that corresponds to df
in the HTML format.
.to_html()
won’t create a file if you don’t provide the optional parameter buf
, which denotes the buffer to write to. If you leave this parameter out, then your code will return a string as it did with .to_csv()
and .to_json()
.
Here are some other optional parameters:
header
determines whether to save the column names.index
determines whether to save the row labels.classes
assigns cascading style sheet (CSS) classes.render_links
specifies whether to convert URLs to HTML links.table_id
assigns the CSSid
to thetable
tag.escape
decides whether to convert the characters<
,>
, and&
to HTML-safe strings.
You use parameters like these to specify different aspects of the resulting files or strings.
You can create a DataFrame
object from a suitable HTML file using read_html()
, which will return a DataFrame
instance or a list of them:
>>> df = pd.read_html('data.html', index_col=0, parse_dates=['IND_DAY'])
This is very similar to what you did when reading CSV files. You also have parameters that help you work with dates, missing values, precision, encoding, HTML parsers, and more.
Excel Files
You’ve already learned how to read and write Excel files with Pandas. However, there are a few more options worth considering. For one, when you use .to_excel()
, you can specify the name of the target worksheet with the optional parameter sheet_name
:
>>> df = pd.DataFrame(data=data).T
>>> df.to_excel('data.xlsx', sheet_name='COUNTRIES')
Here, you create a file data.xlsx
with a worksheet called COUNTRIES
that stores the data. The string 'data.xlsx'
is the argument for the parameter excel_writer
that defines the name of the Excel file or its path.
The optional parameters startrow
and startcol
both default to 0
and indicate the upper left-most cell where the data should start being written:
>>> df.to_excel('data-shifted.xlsx', sheet_name='COUNTRIES',
... startrow=2, startcol=4)
Here, you specify that the table should start in the third row and the fifth column. You also used zero-based indexing, so the third row is denoted by 2
and the fifth column by 4
.
Now the resulting worksheet looks like this:
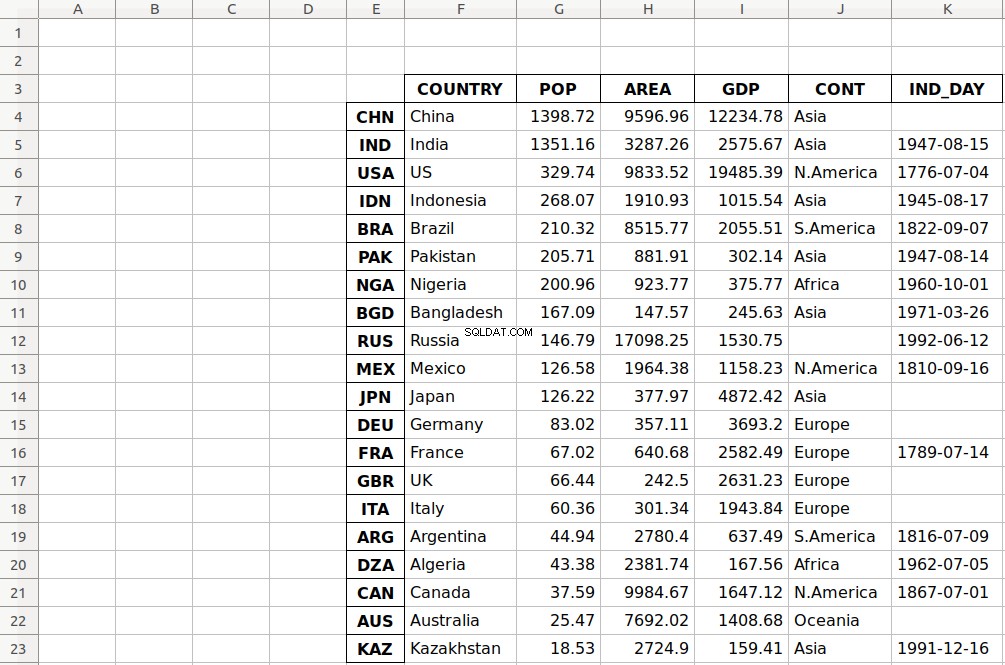
As you can see, the table starts in the third row 2
and the fifth column E
.
.read_excel()
also has the optional parameter sheet_name
that specifies which worksheets to read when loading data. It can take on one of the following values:
- The zero-based index of the worksheet
- The name of the worksheet
- The list of indices or names to read multiple sheets
- The value
None
to read all sheets
Here’s how you would use this parameter in your code:
>>>>>> df = pd.read_excel('data.xlsx', sheet_name=0, index_col=0,
... parse_dates=['IND_DAY'])
>>> df = pd.read_excel('data.xlsx', sheet_name='COUNTRIES', index_col=0,
... parse_dates=['IND_DAY'])
Both statements above create the same DataFrame
because the sheet_name
parameters have the same values. In both cases, sheet_name=0
and sheet_name='COUNTRIES'
refer to the same worksheet. The argument parse_dates=['IND_DAY']
tells Pandas to try to consider the values in this column as dates or times.
There are other optional parameters you can use with .read_excel()
and .to_excel()
to determine the Excel engine, the encoding, the way to handle missing values and infinities, the method for writing column names and row labels, and so on.
SQL Files
Pandas IO tools can also read and write databases. In this next example, you’ll write your data to a database called data.db
. To get started, you’ll need the SQLAlchemy package. To learn more about it, you can read the official ORM tutorial. You’ll also need the database driver. Python has a built-in driver for SQLite.
You can install SQLAlchemy with pip:
$ pip install sqlalchemy
You can also install it with Conda:
$ conda install sqlalchemy
Once you have SQLAlchemy installed, import create_engine()
and create a database engine:
>>> from sqlalchemy import create_engine
>>> engine = create_engine('sqlite:///data.db', echo=False)
Now that you have everything set up, the next step is to create a DataFrame
oggetto. It’s convenient to specify the data types and apply .to_sql()
.
>>> dtypes = {'POP': 'float64', 'AREA': 'float64', 'GDP': 'float64',
... 'IND_DAY': 'datetime64'}
>>> df = pd.DataFrame(data=data).T.astype(dtype=dtypes)
>>> df.dtypes
COUNTRY object
POP float64
AREA float64
GDP float64
CONT object
IND_DAY datetime64[ns]
dtype: object
.astype()
is a very convenient method you can use to set multiple data types at once.
Once you’ve created your DataFrame
, you can save it to the database with .to_sql()
:
>>> df.to_sql('data.db', con=engine, index_label='ID')
The parameter con
is used to specify the database connection or engine that you want to use. The optional parameter index_label
specifies how to call the database column with the row labels. You’ll often see it take on the value ID
, Id
, or id
.
You should get the database data.db
with a single table that looks like this:
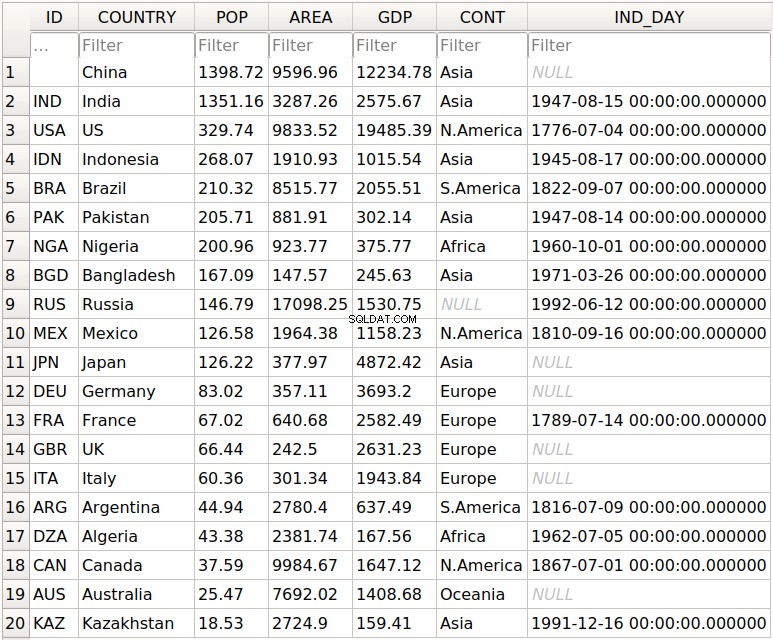
The first column contains the row labels. To omit writing them into the database, pass index=False
to .to_sql()
. The other columns correspond to the columns of the DataFrame
.
There are a few more optional parameters. For example, you can use schema
to specify the database schema and dtype
to determine the types of the database columns. You can also use if_exists
, which says what to do if a database with the same name and path already exists:
if_exists='fail'
raises a ValueError and is the default.if_exists='replace'
drops the table and inserts new values.if_exists='append'
inserts new values into the table.
You can load the data from the database with read_sql()
:
>>> df = pd.read_sql('data.db', con=engine, index_col='ID')
>>> df
COUNTRY POP AREA GDP CONT IND_DAY
ID
CHN China 1398.72 9596.96 12234.78 Asia NaT
IND India 1351.16 3287.26 2575.67 Asia 1947-08-15
USA US 329.74 9833.52 19485.39 N.America 1776-07-04
IDN Indonesia 268.07 1910.93 1015.54 Asia 1945-08-17
BRA Brazil 210.32 8515.77 2055.51 S.America 1822-09-07
PAK Pakistan 205.71 881.91 302.14 Asia 1947-08-14
NGA Nigeria 200.96 923.77 375.77 Africa 1960-10-01
BGD Bangladesh 167.09 147.57 245.63 Asia 1971-03-26
RUS Russia 146.79 17098.25 1530.75 None 1992-06-12
MEX Mexico 126.58 1964.38 1158.23 N.America 1810-09-16
JPN Japan 126.22 377.97 4872.42 Asia NaT
DEU Germany 83.02 357.11 3693.20 Europe NaT
FRA France 67.02 640.68 2582.49 Europe 1789-07-14
GBR UK 66.44 242.50 2631.23 Europe NaT
ITA Italy 60.36 301.34 1943.84 Europe NaT
ARG Argentina 44.94 2780.40 637.49 S.America 1816-07-09
DZA Algeria 43.38 2381.74 167.56 Africa 1962-07-05
CAN Canada 37.59 9984.67 1647.12 N.America 1867-07-01
AUS Australia 25.47 7692.02 1408.68 Oceania NaT
KAZ Kazakhstan 18.53 2724.90 159.41 Asia 1991-12-16
The parameter index_col
specifies the name of the column with the row labels. Note that this inserts an extra row after the header that starts with ID
. You can fix this behavior with the following line of code:
>>> df.index.name = None
>>> df
COUNTRY POP AREA GDP CONT IND_DAY
CHN China 1398.72 9596.96 12234.78 Asia NaT
IND India 1351.16 3287.26 2575.67 Asia 1947-08-15
USA US 329.74 9833.52 19485.39 N.America 1776-07-04
IDN Indonesia 268.07 1910.93 1015.54 Asia 1945-08-17
BRA Brazil 210.32 8515.77 2055.51 S.America 1822-09-07
PAK Pakistan 205.71 881.91 302.14 Asia 1947-08-14
NGA Nigeria 200.96 923.77 375.77 Africa 1960-10-01
BGD Bangladesh 167.09 147.57 245.63 Asia 1971-03-26
RUS Russia 146.79 17098.25 1530.75 None 1992-06-12
MEX Mexico 126.58 1964.38 1158.23 N.America 1810-09-16
JPN Japan 126.22 377.97 4872.42 Asia NaT
DEU Germany 83.02 357.11 3693.20 Europe NaT
FRA France 67.02 640.68 2582.49 Europe 1789-07-14
GBR UK 66.44 242.50 2631.23 Europe NaT
ITA Italy 60.36 301.34 1943.84 Europe NaT
ARG Argentina 44.94 2780.40 637.49 S.America 1816-07-09
DZA Algeria 43.38 2381.74 167.56 Africa 1962-07-05
CAN Canada 37.59 9984.67 1647.12 N.America 1867-07-01
AUS Australia 25.47 7692.02 1408.68 Oceania NaT
KAZ Kazakhstan 18.53 2724.90 159.41 Asia 1991-12-16
Now you have the same DataFrame
object as before.
Note that the continent for Russia is now None
instead of nan
. If you want to fill the missing values with nan
, then you can use .fillna()
:
>>> df.fillna(value=float('nan'), inplace=True)
.fillna()
replaces all missing values with whatever you pass to value
. Here, you passed float('nan')
, which says to fill all missing values with nan
.
Also note that you didn’t have to pass parse_dates=['IND_DAY']
to read_sql()
. That’s because your database was able to detect that the last column contains dates. However, you can pass parse_dates
if you’d like. You’ll get the same results.
There are other functions that you can use to read databases, like read_sql_table()
and read_sql_query()
. Feel free to try them out!
Pickle Files
Pickling is the act of converting Python objects into byte streams. Unpickling is the inverse process. Python pickle files are the binary files that keep the data and hierarchy of Python objects. They usually have the extension .pickle
or .pkl
.
You can save your DataFrame
in a pickle file with .to_pickle()
:
>>> dtypes = {'POP': 'float64', 'AREA': 'float64', 'GDP': 'float64',
... 'IND_DAY': 'datetime64'}
>>> df = pd.DataFrame(data=data).T.astype(dtype=dtypes)
>>> df.to_pickle('data.pickle')
Like you did with databases, it can be convenient first to specify the data types. Then, you create a file data.pickle
to contain your data. You could also pass an integer value to the optional parameter protocol
, which specifies the protocol of the pickler.
You can get the data from a pickle file with read_pickle()
:
>>> df = pd.read_pickle('data.pickle')
>>> df
COUNTRY POP AREA GDP CONT IND_DAY
CHN China 1398.72 9596.96 12234.78 Asia NaT
IND India 1351.16 3287.26 2575.67 Asia 1947-08-15
USA US 329.74 9833.52 19485.39 N.America 1776-07-04
IDN Indonesia 268.07 1910.93 1015.54 Asia 1945-08-17
BRA Brazil 210.32 8515.77 2055.51 S.America 1822-09-07
PAK Pakistan 205.71 881.91 302.14 Asia 1947-08-14
NGA Nigeria 200.96 923.77 375.77 Africa 1960-10-01
BGD Bangladesh 167.09 147.57 245.63 Asia 1971-03-26
RUS Russia 146.79 17098.25 1530.75 NaN 1992-06-12
MEX Mexico 126.58 1964.38 1158.23 N.America 1810-09-16
JPN Japan 126.22 377.97 4872.42 Asia NaT
DEU Germany 83.02 357.11 3693.20 Europe NaT
FRA France 67.02 640.68 2582.49 Europe 1789-07-14
GBR UK 66.44 242.50 2631.23 Europe NaT
ITA Italy 60.36 301.34 1943.84 Europe NaT
ARG Argentina 44.94 2780.40 637.49 S.America 1816-07-09
DZA Algeria 43.38 2381.74 167.56 Africa 1962-07-05
CAN Canada 37.59 9984.67 1647.12 N.America 1867-07-01
AUS Australia 25.47 7692.02 1408.68 Oceania NaT
KAZ Kazakhstan 18.53 2724.90 159.41 Asia 1991-12-16
read_pickle()
returns the DataFrame
with the stored data. You can also check the data types:
>>> df.dtypes
COUNTRY object
POP float64
AREA float64
GDP float64
CONT object
IND_DAY datetime64[ns]
dtype: object
These are the same ones that you specified before using .to_pickle()
.
As a word of caution, you should always beware of loading pickles from untrusted sources. This can be dangerous! When you unpickle an untrustworthy file, it could execute arbitrary code on your machine, gain remote access to your computer, or otherwise exploit your device in other ways.
Working With Big Data
If your files are too large for saving or processing, then there are several approaches you can take to reduce the required disk space:
- Compress your files
- Choose only the columns you want
- Omit the rows you don’t need
- Force the use of less precise data types
- Split the data into chunks
You’ll take a look at each of these techniques in turn.
Compress and Decompress Files
You can create an archive file like you would a regular one, with the addition of a suffix that corresponds to the desired compression type:
'.gz'
'.bz2'
'.zip'
'.xz'
Pandas can deduce the compression type by itself:
>>>>>> df = pd.DataFrame(data=data).T
>>> df.to_csv('data.csv.zip')
Here, you create a compressed .csv
file as an archive. The size of the regular .csv
file is 1048 bytes, while the compressed file only has 766 bytes.
You can open this compressed file as usual with the Pandas read_csv()
funzione:
>>> df = pd.read_csv('data.csv.zip', index_col=0,
... parse_dates=['IND_DAY'])
>>> df
COUNTRY POP AREA GDP CONT IND_DAY
CHN China 1398.72 9596.96 12234.78 Asia NaT
IND India 1351.16 3287.26 2575.67 Asia 1947-08-15
USA US 329.74 9833.52 19485.39 N.America 1776-07-04
IDN Indonesia 268.07 1910.93 1015.54 Asia 1945-08-17
BRA Brazil 210.32 8515.77 2055.51 S.America 1822-09-07
PAK Pakistan 205.71 881.91 302.14 Asia 1947-08-14
NGA Nigeria 200.96 923.77 375.77 Africa 1960-10-01
BGD Bangladesh 167.09 147.57 245.63 Asia 1971-03-26
RUS Russia 146.79 17098.25 1530.75 NaN 1992-06-12
MEX Mexico 126.58 1964.38 1158.23 N.America 1810-09-16
JPN Japan 126.22 377.97 4872.42 Asia NaT
DEU Germany 83.02 357.11 3693.20 Europe NaT
FRA France 67.02 640.68 2582.49 Europe 1789-07-14
GBR UK 66.44 242.50 2631.23 Europe NaT
ITA Italy 60.36 301.34 1943.84 Europe NaT
ARG Argentina 44.94 2780.40 637.49 S.America 1816-07-09
DZA Algeria 43.38 2381.74 167.56 Africa 1962-07-05
CAN Canada 37.59 9984.67 1647.12 N.America 1867-07-01
AUS Australia 25.47 7692.02 1408.68 Oceania NaT
KAZ Kazakhstan 18.53 2724.90 159.41 Asia 1991-12-16
read_csv()
decompresses the file before reading it into a DataFrame
.
You can specify the type of compression with the optional parameter compression
, which can take on any of the following values:
'infer'
'gzip'
'bz2'
'zip'
'xz'
None
The default value compression='infer'
indicates that Pandas should deduce the compression type from the file extension.
Here’s how you would compress a pickle file:
>>>>>> df = pd.DataFrame(data=data).T
>>> df.to_pickle('data.pickle.compress', compression='gzip')
You should get the file data.pickle.compress
that you can later decompress and read:
>>> df = pd.read_pickle('data.pickle.compress', compression='gzip')
df
again corresponds to the DataFrame
with the same data as before.
You can give the other compression methods a try, as well. If you’re using pickle files, then keep in mind that the .zip
format supports reading only.
Choose Columns
The Pandas read_csv()
and read_excel()
functions have the optional parameter usecols
that you can use to specify the columns you want to load from the file. You can pass the list of column names as the corresponding argument:
>>> df = pd.read_csv('data.csv', usecols=['COUNTRY', 'AREA'])
>>> df
COUNTRY AREA
0 China 9596.96
1 India 3287.26
2 US 9833.52
3 Indonesia 1910.93
4 Brazil 8515.77
5 Pakistan 881.91
6 Nigeria 923.77
7 Bangladesh 147.57
8 Russia 17098.25
9 Mexico 1964.38
10 Japan 377.97
11 Germany 357.11
12 France 640.68
13 UK 242.50
14 Italy 301.34
15 Argentina 2780.40
16 Algeria 2381.74
17 Canada 9984.67
18 Australia 7692.02
19 Kazakhstan 2724.90
Now you have a DataFrame
that contains less data than before. Here, there are only the names of the countries and their areas.
Instead of the column names, you can also pass their indices:
>>>>>> df = pd.read_csv('data.csv',index_col=0, usecols=[0, 1, 3])
>>> df
COUNTRY AREA
CHN China 9596.96
IND India 3287.26
USA US 9833.52
IDN Indonesia 1910.93
BRA Brazil 8515.77
PAK Pakistan 881.91
NGA Nigeria 923.77
BGD Bangladesh 147.57
RUS Russia 17098.25
MEX Mexico 1964.38
JPN Japan 377.97
DEU Germany 357.11
FRA France 640.68
GBR UK 242.50
ITA Italy 301.34
ARG Argentina 2780.40
DZA Algeria 2381.74
CAN Canada 9984.67
AUS Australia 7692.02
KAZ Kazakhstan 2724.90
Expand the code block below to compare these results with the file 'data.csv'
:
,COUNTRY,POP,AREA,GDP,CONT,IND_DAY
CHN,China,1398.72,9596.96,12234.78,Asia,
IND,India,1351.16,3287.26,2575.67,Asia,1947-08-15
USA,US,329.74,9833.52,19485.39,N.America,1776-07-04
IDN,Indonesia,268.07,1910.93,1015.54,Asia,1945-08-17
BRA,Brazil,210.32,8515.77,2055.51,S.America,1822-09-07
PAK,Pakistan,205.71,881.91,302.14,Asia,1947-08-14
NGA,Nigeria,200.96,923.77,375.77,Africa,1960-10-01
BGD,Bangladesh,167.09,147.57,245.63,Asia,1971-03-26
RUS,Russia,146.79,17098.25,1530.75,,1992-06-12
MEX,Mexico,126.58,1964.38,1158.23,N.America,1810-09-16
JPN,Japan,126.22,377.97,4872.42,Asia,
DEU,Germany,83.02,357.11,3693.2,Europe,
FRA,France,67.02,640.68,2582.49,Europe,1789-07-14
GBR,UK,66.44,242.5,2631.23,Europe,
ITA,Italy,60.36,301.34,1943.84,Europe,
ARG,Argentina,44.94,2780.4,637.49,S.America,1816-07-09
DZA,Algeria,43.38,2381.74,167.56,Africa,1962-07-05
CAN,Canada,37.59,9984.67,1647.12,N.America,1867-07-01
AUS,Australia,25.47,7692.02,1408.68,Oceania,
KAZ,Kazakhstan,18.53,2724.9,159.41,Asia,1991-12-16
You can see the following columns:
- The column at index
0
contains the row labels. - The column at index
1
contains the country names. - The column at index
3
contains the areas.
Simlarly, read_sql()
has the optional parameter columns
that takes a list of column names to read:
>>> df = pd.read_sql('data.db', con=engine, index_col='ID',
... columns=['COUNTRY', 'AREA'])
>>> df.index.name = None
>>> df
COUNTRY AREA
CHN China 9596.96
IND India 3287.26
USA US 9833.52
IDN Indonesia 1910.93
BRA Brazil 8515.77
PAK Pakistan 881.91
NGA Nigeria 923.77
BGD Bangladesh 147.57
RUS Russia 17098.25
MEX Mexico 1964.38
JPN Japan 377.97
DEU Germany 357.11
FRA France 640.68
GBR UK 242.50
ITA Italy 301.34
ARG Argentina 2780.40
DZA Algeria 2381.74
CAN Canada 9984.67
AUS Australia 7692.02
KAZ Kazakhstan 2724.90
Again, the DataFrame
only contains the columns with the names of the countries and areas. If columns
is None
or omitted, then all of the columns will be read, as you saw before. The default behavior is columns=None
.
Omit Rows
When you test an algorithm for data processing or machine learning, you often don’t need the entire dataset. It’s convenient to load only a subset of the data to speed up the process. The Pandas read_csv()
and read_excel()
functions have some optional parameters that allow you to select which rows you want to load:
skiprows
: either the number of rows to skip at the beginning of the file if it’s an integer, or the zero-based indices of the rows to skip if it’s a list-like objectskipfooter
: the number of rows to skip at the end of the filenrows
: the number of rows to read
Here’s how you would skip rows with odd zero-based indices, keeping the even ones:
>>>>>> df = pd.read_csv('data.csv', index_col=0, skiprows=range(1, 20, 2))
>>> df
COUNTRY POP AREA GDP CONT IND_DAY
IND India 1351.16 3287.26 2575.67 Asia 1947-08-15
IDN Indonesia 268.07 1910.93 1015.54 Asia 1945-08-17
PAK Pakistan 205.71 881.91 302.14 Asia 1947-08-14
BGD Bangladesh 167.09 147.57 245.63 Asia 1971-03-26
MEX Mexico 126.58 1964.38 1158.23 N.America 1810-09-16
DEU Germany 83.02 357.11 3693.20 Europe NaN
GBR UK 66.44 242.50 2631.23 Europe NaN
ARG Argentina 44.94 2780.40 637.49 S.America 1816-07-09
CAN Canada 37.59 9984.67 1647.12 N.America 1867-07-01
KAZ Kazakhstan 18.53 2724.90 159.41 Asia 1991-12-16
In this example, skiprows
is range(1, 20, 2)
and corresponds to the values 1
, 3
, …, 19
. The instances of the Python built-in class range
behave like sequences. The first row of the file data.csv
is the header row. It has the index 0
, so Pandas loads it in. The second row with index 1
corresponds to the label CHN
, and Pandas skips it. The third row with the index 2
and label IND
is loaded, and so on.
If you want to choose rows randomly, then skiprows
can be a list or NumPy array with pseudo-random numbers, obtained either with pure Python or with NumPy.
Force Less Precise Data Types
If you’re okay with less precise data types, then you can potentially save a significant amount of memory! First, get the data types with .dtypes
ancora:
>>> df = pd.read_csv('data.csv', index_col=0, parse_dates=['IND_DAY'])
>>> df.dtypes
COUNTRY object
POP float64
AREA float64
GDP float64
CONT object
IND_DAY datetime64[ns]
dtype: object
The columns with the floating-point numbers are 64-bit floats. Each number of this type float64
consumes 64 bits or 8 bytes. Each column has 20 numbers and requires 160 bytes. You can verify this with .memory_usage()
:
>>> df.memory_usage()
Index 160
COUNTRY 160
POP 160
AREA 160
GDP 160
CONT 160
IND_DAY 160
dtype: int64
.memory_usage()
returns an instance of Series
with the memory usage of each column in bytes. You can conveniently combine it with .loc[]
and .sum()
to get the memory for a group of columns:
>>> df.loc[:, ['POP', 'AREA', 'GDP']].memory_usage(index=False).sum()
480
This example shows how you can combine the numeric columns 'POP'
, 'AREA'
, and 'GDP'
to get their total memory requirement. The argument index=False
excludes data for row labels from the resulting Series
oggetto. For these three columns, you’ll need 480 bytes.
You can also extract the data values in the form of a NumPy array with .to_numpy()
or .values
. Then, use the .nbytes
attribute to get the total bytes consumed by the items of the array:
>>> df.loc[:, ['POP', 'AREA', 'GDP']].to_numpy().nbytes
480
The result is the same 480 bytes. So, how do you save memory?
In this case, you can specify that your numeric columns 'POP'
, 'AREA'
, and 'GDP'
should have the type float32
. Use the optional parameter dtype
to do this:
>>> dtypes = {'POP': 'float32', 'AREA': 'float32', 'GDP': 'float32'}
>>> df = pd.read_csv('data.csv', index_col=0, dtype=dtypes,
... parse_dates=['IND_DAY'])
The dictionary dtypes
specifies the desired data types for each column. It’s passed to the Pandas read_csv()
function as the argument that corresponds to the parameter dtype
.
Now you can verify that each numeric column needs 80 bytes, or 4 bytes per item:
>>>>>> df.dtypes
COUNTRY object
POP float32
AREA float32
GDP float32
CONT object
IND_DAY datetime64[ns]
dtype: object
>>> df.memory_usage()
Index 160
COUNTRY 160
POP 80
AREA 80
GDP 80
CONT 160
IND_DAY 160
dtype: int64
>>> df.loc[:, ['POP', 'AREA', 'GDP']].memory_usage(index=False).sum()
240
>>> df.loc[:, ['POP', 'AREA', 'GDP']].to_numpy().nbytes
240
Each value is a floating-point number of 32 bits or 4 bytes. The three numeric columns contain 20 items each. In total, you’ll need 240 bytes of memory when you work with the type float32
. This is half the size of the 480 bytes you’d need to work with float64
.
In addition to saving memory, you can significantly reduce the time required to process data by using float32
instead of float64
in some cases.
Use Chunks to Iterate Through Files
Another way to deal with very large datasets is to split the data into smaller chunks and process one chunk at a time. If you use read_csv()
, read_json()
or read_sql()
, then you can specify the optional parameter chunksize
:
>>> data_chunk = pd.read_csv('data.csv', index_col=0, chunksize=8)
>>> type(data_chunk)
<class 'pandas.io.parsers.TextFileReader'>
>>> hasattr(data_chunk, '__iter__')
True
>>> hasattr(data_chunk, '__next__')
True
chunksize
defaults to None
and can take on an integer value that indicates the number of items in a single chunk. When chunksize
is an integer, read_csv()
returns an iterable that you can use in a for
loop to get and process only a fragment of the dataset in each iteration:
>>> for df_chunk in pd.read_csv('data.csv', index_col=0, chunksize=8):
... print(df_chunk, end='\n\n')
... print('memory:', df_chunk.memory_usage().sum(), 'bytes',
... end='\n\n\n')
...
COUNTRY POP AREA GDP CONT IND_DAY
CHN China 1398.72 9596.96 12234.78 Asia NaN
IND India 1351.16 3287.26 2575.67 Asia 1947-08-15
USA US 329.74 9833.52 19485.39 N.America 1776-07-04
IDN Indonesia 268.07 1910.93 1015.54 Asia 1945-08-17
BRA Brazil 210.32 8515.77 2055.51 S.America 1822-09-07
PAK Pakistan 205.71 881.91 302.14 Asia 1947-08-14
NGA Nigeria 200.96 923.77 375.77 Africa 1960-10-01
BGD Bangladesh 167.09 147.57 245.63 Asia 1971-03-26
memory: 448 bytes
COUNTRY POP AREA GDP CONT IND_DAY
RUS Russia 146.79 17098.25 1530.75 NaN 1992-06-12
MEX Mexico 126.58 1964.38 1158.23 N.America 1810-09-16
JPN Japan 126.22 377.97 4872.42 Asia NaN
DEU Germany 83.02 357.11 3693.20 Europe NaN
FRA France 67.02 640.68 2582.49 Europe 1789-07-14
GBR UK 66.44 242.50 2631.23 Europe NaN
ITA Italy 60.36 301.34 1943.84 Europe NaN
ARG Argentina 44.94 2780.40 637.49 S.America 1816-07-09
memory: 448 bytes
COUNTRY POP AREA GDP CONT IND_DAY
DZA Algeria 43.38 2381.74 167.56 Africa 1962-07-05
CAN Canada 37.59 9984.67 1647.12 N.America 1867-07-01
AUS Australia 25.47 7692.02 1408.68 Oceania NaN
KAZ Kazakhstan 18.53 2724.90 159.41 Asia 1991-12-16
memory: 224 bytes
In this example, the chunksize
is 8
. The first iteration of the for
loop returns a DataFrame
with the first eight rows of the dataset only. The second iteration returns another DataFrame
with the next eight rows. The third and last iteration returns the remaining four rows.
Nota: You can also pass iterator=True
to force the Pandas read_csv()
function to return an iterator object instead of a DataFrame
oggetto.
In each iteration, you get and process the DataFrame
with the number of rows equal to chunksize
. It’s possible to have fewer rows than the value of chunksize
in the last iteration. You can use this functionality to control the amount of memory required to process data and keep that amount reasonably small.
Conclusione
You now know how to save the data and labels from Pandas DataFrame
objects to different kinds of files. You also know how to load your data from files and create DataFrame
objects.
You’ve used the Pandas read_csv()
and .to_csv()
methods to read and write CSV files. You also used similar methods to read and write Excel, JSON, HTML, SQL, and pickle files. These functions are very convenient and widely used. They allow you to save or load your data in a single function or method call.
You’ve also learned how to save time, memory, and disk space when working with large data files:
- Compress or decompress files
- Choose the rows and columns you want to load
- Use less precise data types
- Split data into chunks and process them one by one
You’ve mastered a significant step in the machine learning and data science process! If you have any questions or comments, then please put them in the comments section below.